Introduction
According Liu and Lee (1), any company can be more integrated and successful in the global marketplace if it has the courage to learn how to improve the management of its supply chain. Supply chain management (SCM) is extensively applied to run and manage business activities in the supply chain. One of the things that need to be considered by a company to improve its supply chain is inventory control, as it plays an important role in supply chain management. Inventory must be controlled competently to meet customers’ demands but failing to manage the demands properly can lead to negative effects such as stockouts, overstocks, and bullwhip effects (2).
Modeling and simulation are the two best-combined methods to be applied to investigate and evaluate the level of inventory management. In addition, an organization with excellent supply chain management has a high potential to minimize or limit the effects of fluctuation in demand. This can be achieved by using different strategies by the company to keep its business position at the maximum competency, despite the variability in demand that occurs in daily life (3).
This study aims to develop and implement different ordering strategies and select the best strategy, together with the goal to improve the business performance throughout the whole supply chain, in terms of improving inventory management and wastage.
Company X, a poultry processing plant, was chosen due to its willingness to provide data in this study in addition to the advantage of its popularity, size, and location. However, understandably it requested anonymity. Company X is part of an international food retail chain that was established in 1987.
The level of wastage is taken into consideration due to the state of the products, which in this case involves poultry, which possesses a short shelf life. This may have an influence on the business profitability in terms of the costs such as obsolescence cost that one needs to investigate. The phenomenon of instability in demand can lead to stockout and overstock issues, which in turn will affect the supply chain inevitably. Buyers may turn to another supplier when stockout occurs, meaning there will be a loss in sales and customers. Furthermore, overstock can increase the holding costs that comprise the costs of rental space, insurance, depreciation, and security.
An industry-standard software, Witness Horizon, is used as a simulation tool, which has the ability to model the processes and operation tasks of the supply chain for Company X. Technically, it has the ability to solve complex business challenges and eliminates the need to carry out physical tests in the real world. The simulation software will develop a predictive virtual representation of Company X’s operations, enabling a high chance to produce certainty and foresight of the operational plans (4).
There are some specific problems and issues encountered by Company X, which were determined by interviewing the manager. According to the manager, one of the main problems encountered by Company X is stockouts where the products are unavailable when the customer’s demand is high. One of the reasons is the change in products’ packaging, which has a high lead time that may cause stockouts. However, every retailer has a similar goal, which is to make and increase profit and also to avoid losing customers concurrently. Reorganizing or redesigning the supply chain may drive the firm toward achieving the aim and be able to minimize any uncertainties, which may occur in the future.
One of the limitations of this study was the lack of opportunity to obtain information from upstream members in the supply chain such as the poultry supplier and processing plant people due to hindrances in gaining access. Therefore, there were several drawbacks in ascertaining the information precisely. However, the beneficial information obtained from the manager of Company X regarding their task operations was ample for the needs to conduct this project.
Literature survey
This research focuses on comparing the forecasting strategies of a poultry SCM regarding the stock inventory levels in a retail environment. Therefore, this study is closely interrelated with a few streams of literature discussed next.
A supply chain encompasses activities linked with the flow and transformation of goods and services from the raw materials stage to the end user (5). A supply chain consists of all parties who are directly or indirectly involved in meeting customers’ demands and includes all functions involved such as marketing, operations, finance, new product development, and customer service (6, 7).
Supply chains are complex and have many uncertainties that exist for many reasons, especially due to a volatile demand (8). To avoid the negative consequences of volatile demand, it is important to incorporate and predict these uncertainties (9). The accuracy of the demand forecast is one of the factors that can contribute to the volatility of demand (10). Demand forecasting is not an easy task, and many companies and forecasters fail to undertake a scientific forecast (11). The biggest problem with demand forecasting is the uncertainty in demand that renders demand forecasting a challenging problem (12).
Demand instability renders forecasting a difficult task and poses excess costs for stockouts, inventory, and capacity utilization (13). The volatility of the demand has been underestimated in supply chain demand forecasting and inventory management. Forecasting to capture the underlying behavior of the volatile demand is crucial to reduce the uncertainty in different levels of the supply chain and to reduce the costs (14).
Forecasting is an activity in the supply chain, where it focuses on maximizing customer value and attaining a sustainable competitive advantage (15). Inaccurate forecasts often cause unnecessary costs in procurement and transportation, manpower, service level, and inventory (16). One of the goals of Company X, like most organizations, is to exceed customers’ expectations in a regular manner by reducing stockouts. Smart forecasting would be the most effective way to satisfy customers’ expectations (17).
A demand forecast may jeopardize the level of success of a supply chain, and to avoid that, one can act according to the laws of forecasting and cultivate them into their business (18). Some observations on the laws of forecasting are given as follows.
Law 1: Forecasts are always wrong
This law explains “how wrong the forecast is” when realizing that there is no forecasting approach that can predict the exact level of future demand. This is due to many factors that would affect the demand. This imprecise method also enables the organization to set a goal to acquire as accurate as possible which has the chance to not give bad impacts on the business when it is within its normal variation. This may be done by using better forecasting strategies.
Law 2: Correct forecast does not mean the method used to forecast is correct
One needs to look at the methodologies they have been using instead of just focusing on the results. The correct forecast could be right once or twice in a while just by luck. It is a situation like flipping a coin where one would be right about half of the time. However, by having a definite methodology to be applied while forecasting, the expected results can be produced consistently.
Law 3: Complicated forecast methodologies can negatively affect the company
Unexpected failures may occur if the complex methods used tend to obscure key assumptions built into the forecast. Contributing knowledge by one’s instincts can help to balance the process of forecasting despite complex methodologies used by supply chain experts.
Law 4: Poor forecasting strategy may not be rectified totally by technology
To make good forecasting, the use of technology can be implemented after a systematic and appropriate strategy has been created. One must think wisely to find ways using technology provided which can improve the bad forecast to a good one; otherwise, it can make the forecast even worse and may affect the whole supply chain.
Methodology
This section explains several techniques, methods, and tools that have been applied in conducting this project. This includes the selection of simulation software, design of the simulation model, and implementation of theories regarding the supply chain.
Witness simulation software
Lanner’s Witness Horizon simulation software was used to model and simulate the activity of SCM. The uncertainty of demand is one of the hurdles in the business world in which the direct application of strategies is not to be advocated or encouraged. It will give a high risk to the organization if one decides to implement strategies in their business without conducting any investigations and evaluations on these strategies in the first place. In this project, accurate models, which represent the behavior of real-world processes, were created statistically by using witness simulation software and were subjected to predictive experimentation. A query of “what if” can be potentially solved if results obtained from the experiments are coherent, without any presence of risks toward the real- life system.
Forecasting techniques
At the end of the supply chain’s activity, customers purchase the goods (poultry) from the retail store, and one can observe the level of demand consequently, whether demand for goods is high or low on that day. The person who is in charge of the store will record all data statistically in order to prepare for the next order. Demands and goods travel in the opposite direction, where demand can be described as the information that travels along the upstream side, while goods or products flow along the downstream side across the supply chain. The movement of both demand and goods (poultry) can be viewed as shown in Figure 1.
The uncertainty in demand can highly affect the replenishment order quantity. Different ordering strategies were implemented in the simulation model to obtain different amounts of inventory replenishment. These alternative ordering methods that were calculated based on the previous week’s demand (PWD), moving average demand (MAD), weighted (forward) 3-point average demand (WAFD), and weighted (backward) 3-point average demand (WABD) can help to determine the order quantity for next days or weeks. By using different ordering strategies, the results in terms of sales and profits would also differ.
The next step in “Methodology” is to model the supply chain after clearly perceiving the idea on how its activities and procedures work. Plus, an interview with the Manager of Company X had been done in obtaining some useful information regarding the ordering data, and all collected data were statistically computed into the simulation model.
There are two phases in forming the supply chain of poultry, where the first phase involves data collection by gathering and measuring primary and secondary sources. The second phase is to build the supply chain process by using Witness Horizon software.
The chicken supply chain
Chicken production takes place in a broiler farm where the hatchery houses a flock of thousands of chickens (Figure 2). To reach market weight, broiler chickens are vaccinated, and they take approximately 7–9 weeks to mature before slaughter (19). The mature chickens are collected and crated to the processing plant where the chickens will be killed, slaughtered, defeathered, eviscerated, and cut. These processes usually take approximately 2–3 days (20). The chickens are then transferred to the distribution center to be kept temporarily for about 18 h to 2 days before being collected at the next location. Next, the chickens are distributed by transporter to each of the retail stores according to the orders made. Finally, all processed chickens in the retail stores are ready to be sold to customers.
Poultry is packed with protein and has a short shelf life. According to Mangaliso (21), poultry quality control is of vital importance in poultry farming due to its raw meat as the end product, which possesses a high possibility to carry disease. Therefore, vaccinating the chickens must be done at the initial phase, in order to avoid any avian diseases. It is important for a company to execute the poultry quality control in order to ensure that they comply with food hygiene requirements and are maintained with impeccable quality before being delivered to the customers. Chickens are products that must be consumed within a short period of time. Hence, the slaughtered and processed chickens must be delivered within a short period of time from the processing plant to the store in order to keep the high quality of the chickens.
Data collection
In the data collection phase, the information was gathered and associated with some parameters such as “Input,” “Activities,” and “Output.” These parameters of the poultry supply chain are displayed in Table 1.
According to the Manager of Company X, batches of chickens are being crated from the broiler farm to the manufacturer for undergoing the process of slaughter, cut, injection, and marination. These processes take 24 h before the marinated chickens are packed and sent to the warehouse. The stocks are then stored in large cold storage in the warehouse at the right temperature in order to keep them fresh. Picking process is executed according to the generated orders and is carried out by the transporter at 6 a.m. This process usually consumes approximately 1 h, and the stocks nominally arrive at the retail store at 7 a.m. with the amount that was ordered. The head office of Company X controls the amount of stock needed to be delivered along with the support from the store manager. A weekly record of a typical amount of chicken demand in a typical retail store is displayed in Figure 3.
In Figure 3, the demand for chickens on weekdays is statistically lower than that on the weekends. This can be seen that on Mondays, there are only approximately 60 units, while on Saturdays, the demand doubled from the amount on Mondays, which is 120 units. The number of orders made by Company X is typically based on the PWD obtained. According to the Manager of Company X, there is a substantial variation in demand volume throughout the year; for instance, during Christmas seasonal time, the demand would be higher than any other month. This is due to the public holiday, high demand on “special menu” that they offer only during Christmas, price promotion, and also the location of Company X as it is located strategically in the city center. However, natural seasonal variation was not considered in this study. Some problems such as high-level stockouts and overstocks may exist during the non-seasonal time, and these issues must be drawn special attention to in order to reach a high level of profitability and to lower costs. These issues have been arithmetically computed, observed, analyzed, and resolved using a competent simulation software, which will be explained in the next part.
Simulation model
Witness Horizon was used to model the supply chain’s activities for Company X. The evaluation of the impact toward Company X after implementing different ordering strategies was the main purpose to build this model, as it also provides the comprehension to the users on how a business operates in actual time. The simulation software was used as a tool to conduct experimental analysis and also to investigate the potential improvements of the supply chain of Company X. There were different stages of operations involved in the supply chain model such as manufacturing, warehousing, picking and delivering, stock shop, and customer demand. The modeling feature is classified into the following components: Elements (entities, queues, and activities), Input or Output Rules, Match/Any Rules, and Distributions. Figure 4 shows a finalized chicken supply chain in Witness Horizon with its element flow.
Next, the base time units were converted from the default minutes to days, and there were some descriptions of model details that have been created in the supply chain model. Entity element such as “Daily Signal” was created as it updates the Current Day and Day Number in the model during simulation, and entity “Order “ represents a request to replenish stock. “Stockshop” and “Warehouse” are examples of queues that hold the products. Then, they are required by next activities such as “Sales Counter” (the place triggering customer’s demand) that pulls the goods from “Stockshop” and the “Picking” station that pulls the goods from “Warehouse.” Three distributions were created as each distribution has different demand quantities and probabilities. These three distributions were defined as DailyDemand1, DailyDemand2, and DailyDemand3. DailyDemand1 indicates the assumptions of the demand raised on Mondays and Tuesdays (approximately 60 to 80 units), DailyDemand2 indicates the demand raised on Wednesdays and Thursdays (approximately 70 to 100 units), and DailyDemand3 indicates the demand raised on Fridays, Saturdays, and Sundays (approximately 90 to 120 units).
A management information system (MIS) and a finance information system (FIS) were created, and they acted as a dashboard that contains statistics results from the analysis of the constructed model. The results in MIS and FIS presented in a tabular form were updated dynamically during the simulation and display 60 days of continuous operations.
The sample of results displayed in the form of MIS and FIS is shown in Figure 5, where MIS contains the management data from column 1 to column 8, while FIS contains the financial data that can be seen from column 9 to column 11. Column 12 contains the wastage, which is also categorized under MIS. Table 2 details the descriptions for each column of MIS and FIS.
Poultry is a perishable product that has an extremely short shelf life where it can become unsafe to consume if it is not kept refrigerated at its lowest required temperature. Unlike frozen food that has a long shelf life, one must take into account how to manage the level of inventory and ordering strategies for short shelf-life products, like chickens, in avoiding any additional costs. Hence, a column of “Disposed” was devised and created in order to observe and record the number of spoiled and unusable chickens, which may be affected by the firm’s inventory level. In the simulation, a “dwell buffer” (with Max_Delay option) was used in order to calculate the maximum time that each part or stock should spend in the buffer as it enters the buffer. If the stock is still in the buffer after the (fixed) time has elapsed, the stock will be automatically removed. This means that the chickens that are still in the buffer for 5 consecutive days (end of day 5) will be disposed in the early morning on day 6. The Manager of Company X stated again during the interview that chickens have the ability to maintain their freshness for 5 days maximum as long as they are placed in a room with a required fixed low temperature. This is also due to the sophisticated design of the marinade recipe that can help to increase the shelf life of the chickens, in addition to the proper fine packaging.
Improvement strategies
Three phases of “improvement methods” were devised in the simulation process in order to see how sensible and realistic the results of the firm can produce: initial improvement phase, second improvement phase, and final improvement phase.
The first phase is the initial improvement phase, where improvement in this phase refers to an observation of the performance that can be improved by using different ordering strategies such as PWD, MAD, WAFD, and WABD. It was conducted solely to observe, analyze, and compare the four results obtained and select which one was preferable. The result might show either an improvement or deterioration toward the level of profitability, inventory, and wastage during the 60 operating days.
Second improvement phase introduced a “Reduce Overstocking” method, which was conducted after the initial improvement phase. This method was believed to be a reliable approach that can reduce the level of overstocks and the number of spoiled products (which might occur in initial improvement phase). This is due to an assumption that the results in the initial phase might show the number of overstocks accumulating consecutively and would produce a high number of wastages. These problems could be solved or avoided after implementing the “Reduce Overstocking” method.
The third phase is the final improvement phase, which was devised to be a method that might produce the most- improved results as compared to the results in previous (initial and second) phases. This was conducted after the second improvement phase, and better results were produced positively in this final phase in terms of improving inventory and wastage.
After obtaining the results from the simulation, which was run for 60 days, a comparison of different ordering strategies was performed, which led to evaluating and selecting the most beneficial strategy that can improve the performance of the supply chain. Once the comparison had been made, experimentations using Simple Experiment Mode in Witness on the randomness and variability were taken into consideration within the simulation. This was done by setting a number of replications that would run the simulation multiple times with different random numbers. The idea behind this experimentation is that these random numbers generate variability in the results that can develop one’s confidence level in obtaining the most accurate and sensible result through the experimentation. The experimentations were done in the phases of final improvement in each strategy.
Results and experimentation
This section will demonstrate the results obtained from the scrutinized simulation test. An evaluation of the performance of the chicken supply chain was conducted, and strategies related to the ordering of inventory replenishment were devised. Four different strategies were introduced and simulated for 60 days, which are based on the demand of WABD (Strategy 1), WAFD (Strategy 2), MAD (Strategy 3), and PWD (Strategy 4).
In addition, three improvement phases were devised in order to achieve the final-improved result (which had been explained in the “Methodology” section), namely, the initial improvement phase, second improvement phase, and final improvement phase. The simulation plots and results are displayed separately in each strategy. Typically, the figures show the demand versus inventory plot after 60 days of simulation.
Strategy 1: Initial improvement phase in WABD strategy
The quantity of today’s order is based on the forecasting of WAFD. The first order made was on the fourth day, and the last or most recent value (in this case, demand on the third day) consists of the highest weighting as compared to the two other values on consecutive days before (on the first and second day). Moreover, it is assumed that the number of orders made on days two and three will be according to yesterday’s number of demand. In this phase, the calculation of WAFD was computed in the simulation model, and Figure 6A shows the results for demand and inventory.

Figure 6. (A) Demand versus inventory plot after 60 days of simulation (Initial: WABD). (B) Demand versus inventory plot after 60 days of simulation (Second: WABD). (C) Demand versus inventory plot after 60 days of simulation (Final: WABD).
From Figure 6A, the quantity of demand and inventory rose and fell within 60 days of simulation. The level of inventory almost reached the highest level of 200 units, which usually occurred virtually on Wednesdays and Thursdays. This is due to poor management of inventory control, which is caused by previous days’ overstocks carried forward to the next day. In other words, this situation is possibly caused by the excess amount of previous consecutive overstocking patterns. However, there was a small number of customers, which is approximately 7%, who were unable to purchase the products due to lack of stock, yet opportunely shows the stocks were 93% available when customers needed them within the 60 operating days. The method used in this phase has shown that it is a reliable strategy to be used in order to sustain the level of stock availability or provide low chances to have stockout. The level of product waste is quite high, that is, approximately 243 units were disposed within 60 days. The inventory level remained high, even though it fluctuated continually. To minimize the high level of inventory, the method of “Reduce Overstocking” was conducted in the second phase.
Strategy 1: Second improvement phase in WABD strategy
From Figure 6B, the quantity of demand and inventory was still fluctuating consistently over 60 days of simulation. However, the level of inventory was evidently minimized after implementing the additional method of “Reduce Overstocking.” In fact, it can be seen from Figure 6B that the stock levels in the inventory did not reach beyond 150 units, and the stipulated difference of amount between demand and number of stocks was not as high as the result in the initial phase. This brings to the essential point where the rate of the number of stocks ordered that led to overstocking and the number of products’ waste were substantially reduced. However, the number of stockouts dramatically increased by 50%. However, facing a rapid increase in stockouts and a potential decline in profitability needs to be recuperated, and this can be done by using another additional method, that is, the final improvement phase.
Strategy 1: Final improvement phase in WABD strategy
This phase shows (Figure 6C) the final refinement in terms of the level of inventory, stockouts, and product waste within 60 days. From Figure 6C, the improvement can be seen; for instance, on days 43 to 45 and also on days 56 to 59, the condition of the inventory (green line) is just above the demand level (red line). This condition indicates the level of stock is not excessive and able to meet customer needs consequently. In addition, the level of inventory was once more reduced together with the number of stocks. Moreover, there was a sharp decrease in the number of spoiled products.
Strategy 2: Initial improvement phase in WAFD strategy
Figure 7A shows the fluctuation of demand and inventory levels within 60 operating days. By using the strategy of WAFD, the amount of inventory increased significantly from day 1 to day 10 and dropped slowly at the end of day 10 before the inventory level went up again at the end of day 14. As can be seen, the level of inventory is undulated continually within 60 operating days. However, the level of stock did not go beyond 150 units on each of the days, which is better and shows no high extreme amount of stock as compared to the initial improvement WABD strategy, where it exceeded 150 units. The product waste is moderately high, approximately 175 units. The company experienced an infrequent stockout condition where it shows only 5% of customers who are unable to purchase the product due to a lack of stocks within 60 days. The inventory level remained higher than the demand itself. This could be minimized by using the second phase method of “Reduce Overstocking,” which is discussed next.
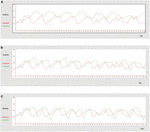
Figure 7. (A) Demand versus inventory plot after 60 days of simulation (Initial: WAFD). (B) Demand versus inventory plot after 60 days of simulation (Second: WAFD). (C) Demand versus inventory plot after 60 days of simulation (Final: WAFD).
Strategy 2: Second improvement phase in WAFD strategy
After implementing the method of “Reduce Overstocking,” it can be seen from Figure 7B that the green line was going toward a lower position than that in the previous phase. This indicates that the quantity of overstocks in the inventory was well decreased, resulting in an improvement from 45 overstocking level to 30% overstocking level. However, the method used has led to over-minimization, which sometimes causes the green line (inventory) to appear below the red line (demand). This has produced another problem, which is an increase in stockouts, and the company lost their customers by 6% as compared to the previous strategy. On the bright side, no products were needed to be disposed in this phase of the strategy.
Strategy 2: Final-improvement phase in WAFD strategy
From Figure 7C, there are nearly six sharp troughs, which usually occurred on either Wednesdays or Thursdays. This indicates that the inventory encountered the stockout condition at its most critical point six times within 60 operating days. The inventory level decreased by 1%. Moreover, the rate of stockout increased from 11 to 15% and the number of waste products existed which shows that this final-improved strategy is unlikely to be implemented in order to enhance or improve the process of supply and demand.
Strategy 3: Initial improvement phase in MAD strategy
Figure 8A shows a big gap between the line of inventory and the line of demand. This was probably due to the safety stock issue and the effect of fluctuation in demand that the company had encountered. By using this initial phase strategy, it seems the occurrence of stockouts rarely occurred within the 60 days during which the rate of customers unable to purchase the products was only 3%. However, excessive safety stocks led to high amount of products that would be disposed due to their short shelf life.
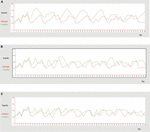
Figure 8. (A) Demand versus inventory plot after 60 days of simulation (Initial: MAD). (B) Demand versus inventory plot after 60 days of simulation (Second: MAD). (C) Demand versus inventory plot after 60 days of simulation (Final: MAD).
Strategy 3: Second improvement phase in MAD strategy
Figure 8B illustrates the strategy of the second improvement phase using MAD. It is obvious that the level of inventory was noticeably minimized. Despite the occurrence of the shortfall, the lowest quantity of stocks in the inventory was virtually the same or higher than the lowest quantity of demand within 60 days. The amount of spoiled products was rapidly reduced by 123 units. The rate of stockout was slightly increased by 6 and 92% of the demand was successfully ordered.
Strategy 3: Final improvement phase in MAD strategy
Figure 8C illustrates that the gap between the level of inventory and demand was small and that most of the time, the stocks in the inventory were just sufficiently enough when the customer needed them. The quantity of overstocking products in the inventory had reduced by 22%, despite the occurrence of continual fluctuations. The quantity of product wastage had also dropped to 29 units, and 92% of the demand was successfully ordered.
Strategy 4: Initial improvement phase using PWD
By using this strategy, shown in Figure 9A, there was a huge gap between the level of inventory and demand at the end of week 8 due to an excessive amount of stocks, which increased consecutively (in an average of 5 days), and also due to the demand being consistently low on that week. The level of wastage was the highest as compared to other phases, where it disposed 230 units within 60 days. This was due to the short shelf life of the products together with constant accumulated stocks in the inventory, especially at week 8 and week 9. However, the stockout situation barely occurred, and it was only 2% of customers who are unable to purchase the products.

Figure 9. (A) Demand versus inventory plot after 60 days of simulation (Initial: PWD). (B) Demand versus inventory plot after 60 days of simulation (Second: PWD). (C) Demand versus inventory plot after 60 days of simulation (Final: PWD).
Strategy 4: Second improvement phase using PWD
Figure 9B shows the obvious level of inventory that has been minimized by using the second improvement method. Fluctuations in demand and inventory level still occurred, yet the gap between those lines was not enormous. The two critical situations occurred in week 7 where the level of inventory rapidly increased at the end of Saturday and dropped sharply from the end of Monday to Wednesday. However, stock ordering was made at the end of Tuesday, and there was an inventory replenishment at the beginning of Thursday. Wastage levels had decreased drastically by over 90% due to the reduction in overstocking level.
Strategy 4: Final-improvement phase using PWD
From Figure 9C, the pattern of the graph was fairly similar to the second improvement phase where both demand and inventory levels are undulated through the days. On the gloomy side, a rapid decline in the inventory level had occurred from days 23 to 25 that led to a critical point on day 25 itself, which is due to an increase in demand within that week. The rate of losing customers due to stockouts also increased by 6%. This strategy might not be sensible enough if one focuses on improving its profitability.
Discussion
In this section, the evaluation of the results obtained is conducted, along with a discussion and analysis of some approaches that shall improve the supply chain performance of Company X, theoretically.
The study of a chicken supply chain has been carried out in collaboration with Company X. Different types of ordering strategies have been devised and applied to different simulation tests, for example, strategies based on WABD, WAFD, MAD, and PWD. Each strategy has produced different results, where one of the strategies is selected as the most preferable strategy that shall be proposed and used by Company X in order to improve its supply chain performance.
Graphs from all different strategies have shown that both demand and inventory levels fluctuated from day 1 until day 60. There were huge gaps between demand and inventory levels in each initial improvement phases, but majority of the gaps are shrinking toward the final improvement phases. This is due to the efficacy of the methods used in each phase that helped to reduce the size of gaps and produced the same level of quantities virtually. However, there were points where the inventory level does not match the customer’s demand level, which would lead to either stockout or overstock. The most desirable condition is when the green line (inventory) appears above the red line (demand) carrying some safety stock. Unfortunately, this did not happen smoothly, and there were also few large unexplained drops in inventory level which were probably encountered due to some issues such as errors in forecasting, lead time variability, or even inflated order. Nevertheless, a graph of final improvement phase in the MAD strategy having neither large spikes nor drops as compared to other strategies shows the most potential. This indicates that the MAD strategy shall be implemented by the firm in order to improve its supply chain performance.
It has been discovered that the most preferable strategy that can be implemented by Company X is the ordering strategy based on MAD (in final improvement phase). The results in MAD have shown a positive and reliable improvement in terms of its level of inventory, as well as the wastage level. The best strategy was chosen based on the evaluation and analysis conducted throughout every phase (initial, second, and final improvement phases) in each strategy. For instance, in the initial improvement phase, the MAD strategy gained the second lowest rate of “ordered led to overstocking,” after the PWD strategy (difference by 2% of overstocking). However, the MAD strategy possesses the lowest amount of wastage as compared to the other three strategies in the initial phase. This indicates that the company possesses less burden to cover the obsolescence cost, in other words, less encountering the holding cost.
By using WABD strategy, Company X has to endure the burden of having the highest amount of overstocking and wastage. It is very clear from the results obtained earlier that the rate of “ordered led to overstocking” in the WABD strategy is the highest, due to an excessive amount of previous consecutive overstocking patterns. This situation will be explored in the next section.
Table 3 shows a combined data of initial improvement phases in four strategies (WABD, WAFD, MAD, and PWD) from day 22 to day 40, which was extracted from each MIS.

Table 3. Number of overstocking products in initial improvement phases for all four strategies (from MIS).
As shown in Table 3, the quantity of overstocking products is accumulating consecutively in every ordering strategy. The level of wastage in WABD has the highest quantity due to the uncontrolled number of overstocking products. This situation is due to the supply exceeding the demand. This also occurred along with the summation of today’s amount of supply and the previous day’s overstocking products, which happened consecutively. In the initial improvement phases (for all four strategies), the more frequent the products being supplied (with high quantity consecutively), the higher the chance for the products to be overstocked, which would occur continually, due to demand uncertainty. This also shows that the quantity of overstocking has affected the level of wastage consequently. For example, orders are made in the range of the same (high) quantity, every day, for a week. Then due to demand uncertainty, overstocks occur in each of those 7 days, consecutively, which will result in the likelihood of some unusable products (which have lost their value after a specific date) to be disposed on day 8. This problem obviously occurred in the initial phase of all four strategies, but the WABD strategy was the most unfavorable one to be chosen among others. Second improvement phase was developed for the purpose of reducing the number of supplies, which has successfully resulted in reducing the level of overstocking products in the inventory. It can be seen that the number for today’s order has been reduced dramatically after it had been triggered by the overstocking situation, which happened consecutively in the previous 3 days or more in the inventory. It also resulted in an overall small quantity of disposed products within 60 operating days.
All retail stores, especially those involved in fresh food industries, are against the fact of them having and holding the inventory with excessive overstocking products consecutively. Unlike frozen food, Company X concerns about fresh food that has an extremely short shelf life, which must be sold immediately once it reaches the store. However, the drawbacks after implementing this strategy (in the second improvement phase) are that the number of stockouts has increased than before.
In addition, when products are not available or ample in the inventory, backorder and lost sales costs might be incurred. Company X has to cover the backorder costs, which typically include extra costs for administration or expediting material handling and transportation. In fact, lost sales costs of inventory are also incurred due to missing out on sales, which leads to losing gross profit. Plus, stockout also may incur a less tangible cost which Company X might encounter in a loss of customer goodwill. All these costs affect negatively monetary sales and potential profitability.
One of the desirable outcomes was to improve the profitability of the firm. However, with stockouts and waste, it was realized that potential profits have diminished from using the four strategies (WABD, WAFD, MAD, and PWD), particularly during the second improvement phase due to cost incurrence. To address these issues, the final improvement phase was developed to minimize the wastage level. The strategy devised was to order 20% more unit of products for the following day after realizing the fact that the stockout level has been in critical condition for the past few consecutive days (2 days or more). However, the 20% extra units will not be ordered if the event of stockouts did not occur consecutively in the past few days, where ordering more (when the stock level is already high) would cause overstock; 20% or 0.2 is an optimum coefficient chosen due to its realistic outcome (after a number of simulations were conducted using different coefficients, where the results obtained are not preferable as of using 0.2). It is believed that the higher the ratio used (more than 0.2), the lower the chance to have desirable profits. Furthermore, the strategy used in the final improvement phase has ensured the adequacy amount of the safety stock level that has the ability in helping to have as much information and control as possible over each inventory item, and always be in a sustainable manner. This may lead to a high possibility to prevent stockout and, at the same time, avoid overstock.
An organization has the ability to improve its SCM by finding the right balance between minimizing its stocks and being able to meet the order demands. Proper demand forecasting can add value to any organization if the approaches and efforts used are well-organized systematically and act according to the laws of forecasting. Leadership engagement and excellent communication must be well-executed to align the internal and external stakeholders to achieve their mutual goals. Finally, using advanced planning and scheduling can support planners in making better decision based on the right information and helps businesses to optimize the business operation.
Conclusion
Supply chain management is very important as it is an integral part of most businesses. The higher the quality of an SCM, the higher the chance for a company to succeed and to meet customers’ needs. To improve the SCM of Company X, some ordering strategies have been successfully explored and developed such as the strategy based on PWD, MAD, WAFD, and WABD. All four strategies have successfully been analyzed under three improvement phases, namely, the initial, second, and final improvement phases. Witness Horizon was well utilized as a simulation tool that modeled and examined the supply chain for Company X and also produced reliable results. It was found that using the final improvement of MAD strategy would be the most preferable strategy that can be used in order to improve the business performance or SCM for Company X. This is due to the results obtained, which indicates a high probability of improving the level of the company’s profitability, inventory, and wastage. The results obtained from this research depict that discrete event simulation can be a very useful technique for the simulation of demand forecasting using various time series based forecasting methods in chicken processing supply chains and making inventory decisions based on simulated forecasts. This research demonstrates that simulations can generate randomness and uncertainty scenarios associated with the demand patterns in chicken processing supply chains which can help decision-makers to make strategic inventory decisions based on what-if type analysis.
Author contributions
BA conceived the idea, developed the simulation, and drafted the manuscript. SK and ML contributed, refined, and enhanced the manuscript. All authors have read and agreed to the published version of the article.
Declaration of interests
The authors declare that they have no known competing financial interests or personal relationships that could have appeared to influence the work reported in this study.
Funding
This research received no specific grant from any funding agency in the public, commercial, or not-for-profit sectors. However, Manchester Metropolitan University is acknowledged for supporting the research.
Acknowledgments
The authors acknowledge the contributions of specific colleagues, institutions, or agencies that aided their efforts.
References
1. Liu C, Lee M. Integration, supply chain resilience, and service performance in third-party logistics providers. Int J Logist Manag. (2018) 29:5–21.
2. Zhang H, Wang Z, Hong X, Gong Y, Zhong Q. Fuzzy closed-loop supply chain models with quality and marketing effort dependent demand. Expert Syst Appl. (2022) 207:118081.
3. Ong J, Latif M, Kundu S. Exploiting witness simulation for SCM. Int J Res Manag Sci Technol. (2014) 2:103–9.
4. The Lanner Group.Predictive simulation modelling software. (2022). Available online at: https://www.lanner.com/ (accessed 02 October 2022).
5. Gao X, Cao C. A novel multi-objective scenario-based optimization model for sustainable reverse logistics supply chain network redesign considering facility reconstruction. J Clean Prod. (2020) 270:122405.
6. Chopra S, Sodhi M, Lucker F. Achieving supply chain efficiency and resilience by using multi-level commons. Decis Sci. (2021) 52:817–32.
7. McKay A, Chittenden R, Hazelhurst T, de Pennington A, Baker R, Waller T. The derivation and visualization of supply network risk profiles from product architectures. Syst Eng. (2022) 25:421–42.
8. Abolghasemi M, Eshragh A, Hurley J, Fahimnia B. Demand forecasting in the presence of systematic events: cases in capturing sales promotions. Int J Prod Econ. (2020) 230:107892.
9. Christopher M, Holweg M. Supply chain 2.0 revisited: a framework for managing volatility-induced risk in the supply chain. Int J Phys Distrib Logist Manag. (2017) 47:2–17.
10. Guo F, Diao J, Zhao Q, Wang D, Sun Q. A double level combination approach for demand forecasting of repairable airplane spare parts based on turnover data. Comput Industr Eng. (2017) 110:92–108.
11. Makridakis S, Spiliotis E, Assimakopoulos V. The m4 competition: results, findings, conclusion and way forward. Int J Forecast. (2018) 34:802–8.
12. Syntetos A, Babai Z, Boylan JE, Kolassa S, Nikolopoulos K. Supply chain forecasting: theory, practice, their gap and the future. Eur J Operat Res. (2016) 252:1–26.
13. Christopher M, Holweg M. Supply chain 2.0: managing supply chains in the era of turbulence. Int J Phys Distrib Logist Manag. (2011) 41:63–82.
14. Chen JY, Dimitrov S, Pun H. The impact of government subsidy on supply chains sustainability innovation. Omega. (2019) 86:42–58.
15. Dong L, Tang SY, Tomlin B. Production chain disruptions: inventory, preparedness, and insurance. Prod Operat Manag. (2018) 27:1251–70.
16. Torkul O, Yilmaz R, Selvi I, Cesur M. A real-time inventory model to manage variance of demand for decreasing inventory holding cost. Comput Industr Eng. (2016) 102:435–9.
17. Antucheviciene J, Jafarnejad A, Amoozad Mahdiraji H, Razavi Hajiagha SH, Kargar A. Robust multi-objective sustainable reverse supply chain planning: an application in the steel industry. Symmetry. (2020) 12:594–617.
18. Robertson G. Lawsofforecastingtohelpbrandleaders[blog] Beloved brands. (2013). Available online at: http://beloved-brands.com/2013/10/06/forecasting (accessed 02 October, 2022).
19. Martin.How long does it take for broiler chickens to be ready for the market. (2013). Available online at: https://articles.extension.org/pages/67129/how-long-does-it-take-for-broiler-chickens-to-be-ready-for-the-market (accessed 02 October, 2022).
20. Capkova N. Chicken Run - The poultry supply chain. (2015). Available online at: https://prezi.com/vzhao5pfbars/chicken-run-the-poultry-supply-chain/ (accessed 02 October, 2022).
21. Mangaliso M. How products are made: Chicken. (2012). Available online at: http://www.madehow.com/Volume-5/Chicken.html (accessed 02 October, 2022).